AIAugSurgery
Mixed reality systems for endoscopy surgery.
計畫說明 計畫期程:2018.7 ~
內視鏡微創手術(包含腹腔鏡,子宮內視鏡,膝肩內視鏡等)具有傷口小,失 血量較少,復原快以及有效使用醫療資源的特性。但是,手術的成敗與醫師的 熟練程度高度相關。手術過程中,醫師必須仰賴視角有限的視訊鏡頭了解手術 器械以及目標器官在體內的位置,手術有相當的難度。目前的內視鏡手術實施 方式,需要醫師一邊觀看螢幕上的內視鏡影像,一邊觀察手上器械的位置下進 行,影響開刀的準確性。本計劃將利用團隊過去在即時嵌入式系統影像處理以 及使用類神經網路的研究經驗,發展提供微創手術使用的混和實境體內定位與 導航技術,在手術的過程中,可以同時觀看內視鏡影像,即時醫療資訊以及手 部動作。
混和實境內視鏡導航的目的在內視鏡的影像上,經由影像辨識技術,取得影像 中的器官種類以及特徵點後,使用同步定位與地圖構建(SLAM)技術,取得該 臟器以及手術器械在體內的位置。取得之特徵點則可提供三維模型定位點,並 依此將三維模型套疊在即時影像上,包含動脈血管,靜脈血管,目標腫瘤位置 等,提供醫師精準與豐富的即時資訊。
本計劃將在四年內研究以下幾項重要議題:(1)非剛性物體辨識:研究團隊過 去對於汽車,機器人,場地線等剛性物體的即時辨識,都已經具有成熟的技術, 但是,非剛性物體的辨識與剛性物體辨識有相當大的差異。其主要的挑戰在於, 非剛性物體的特徵點會因為形變或是遮蔽而無法辨識,此外,也特徵點之間的 空間關係也會因為手術操作而改變,不易辨識。(2)體內定位即時排程分析: 本系統的硬體架構將使用嵌入式與雲端系統共構的架構,以提昇使用者經驗, 降低能源使用同時取得高精確度的結果。但是,在此一架構中,計算資源(包 含嵌入式系統上的CPU/GPU以及雲端系統的CPU/GPU)的分配會影響是否能提供 即時定位與即時混和實境合成。(3)半監督式個人化器官模型: 本計畫將使用 生成式方法(Generative Model)為基礎,進行即時器官辨識與定位,因此,模 型的準確性會直接影響辨識的準確性。雖然,人類器官具有高度的共通性,但 是,微創手術的適用對象以病患居多,其器官外觀可能會因為為病變而與健康 的器官有顯著的差異,而此一差異會對辨識的準確度有顯著的影響。因此,我 們將使用半監督式學習的方式為每位患者建構器官辨識模型,作為辨識的基礎, 以提昇辨識的正確率。
本計劃的目標在研究與開發提供即時影像辨識與體內導航的嵌入式混和實境系 統之非剛性物體辨識,體內定位即時排程分析,以及半監督式個人化器官模型 建立等關鍵技術。基於研究團隊在嵌入式系統影像辨識,異質計算平台即時運 算等技術的基礎,可順利完成此一計畫目標。
Project Description Project period: 2018.7 ~
Endoscopic surgery (including orthopedic surgery, endodontic surgery, endoscopic endonasal surgery, and endoscopic spinal surgery) has many benefits. Small incisions, less pain, lesser duration of hospital stay (Approximately 1/2 that of open surgery), rapid recovery to normal active life and reduced blood loss are few examples. However, it also has several shortcomings. First of all, it requires one or more years to train the physicians. Endoscopic surgery requires the physicians to handle endoscopy and instruments at the same time in order to monitor the location of instruments and organs simultaneously. Current equipments require the physicians to watch a monitor to view the images from endoscopy and lower his eyesight to view the hand pose while handling the instruments. As a result, there are many errors during the surgery. In this project, we plan to develop the algorithm and software to enable mixed reality endoscopy. With this technology, the physician can wear the lightweight glass to view the images from endoscopy, hand movements, and medical information (including artery, vein, targeted tissue which are usually hidden).
The goal of mixed reality in-body navigation technology is to use object recognition technology to identify the organs and tissues from endoscopy images. The recognized features will be extracted to match with computed 3D mesh, which provides artery, vein, targeted tissue, to be overlaid on the endoscopy images. With these information, the physicians can have real-time images from endoscopy images and the medical information collected from earlier checkups and instructions for the surgery.
To achieve the aforementioned goals, the project will target the following challenges in four year periods. (1) Non-rigid object recognition: the research team has been working the rigid object cognition, including vehicle, traffic sign, and robots in last few years and has outstanding achievements. However, the human organs and tissues are not rigid objects and have significantly different features. The size, form, and spacial relations of features of non-rigid object will change from time to time. Hence, it requires different techniques for accurate recognition. (2) Schedulability analysis for in-body localization and object recognition: to support endoscopic surgery, the process of mixed reality have to provide real-time response. However, the hardware platforms targeted in this project have limited computation resources in order to support high mobility and long duration. Hence, the computation resources must be allocated and scheduled to meet real-time constraints. We will target the multi-core CPU, GPU, and AI processors to conduct the schedulability analysis and resource allocation. (3) Semi-supervised incremental learning for personalized classification models. The same organ of different persons may look similar overall. However, in this application, the patients are usually picked and his/her organs are not likely as same as health ones. While using the same classification model to recognize the organ, the accuracy will be low. Hence, we will develop the method and algorithm to enable semi-supervised incremental training to construct the personalized classification model.
The research team has been working on real-time object recognition and SLAM for robots in last few years. The knowledge in these two fields can be extended to tackle the challenges targeted in this project.
Project progress summary
- Hand gesture recognition
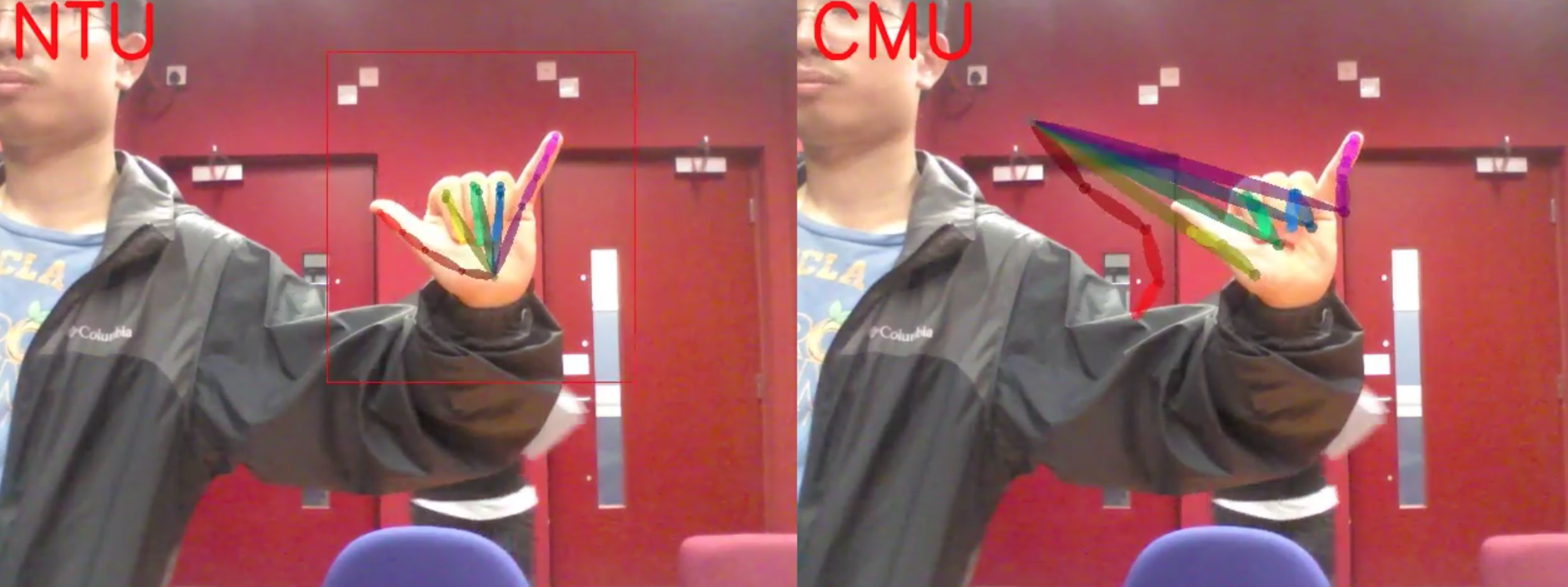
- Semantic segmentation for Endoscopy and robotic surgery images
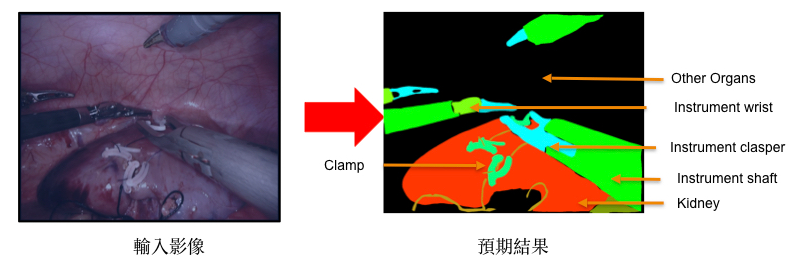
- Navigation for Endoscope
- Specular Removal for Endoscopic Video